(Essay found in Nesselroade & Grimm, 2019; pgs. 236 – 237)
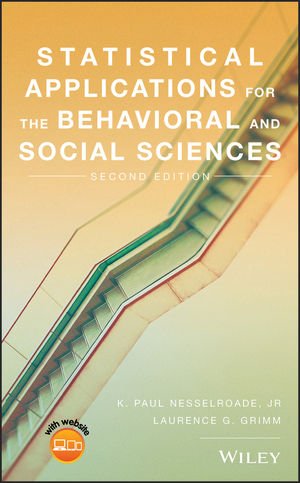
If there is a “ground zero” for the current reproducibility crisis in the social, behavioral, and medical sciences, it may be found in the personhood of John Ioannidis, Professor of Medicine and of Health Research and Policy at the Stanford University School of Medicine. In 2005, he published an article in PLoS Medicine entitled, “Why Most Published Research Findings are False.” As one might imagine, this article created a firestorm of controversy as well as an avalanche of articles reacting to this claim; some supporting (e.g., Freedman, 2010), some critiquing (e.g., Leek & Jager, 2017). As a result, Ioannidis is currently one of the most-cited scientists in the world.
Several of the points Ioannidis makes in the paper involve misunderstanding the perils of Type I errors. The Type I error rate is an accurate reflection of the risk involved in rejecting a singular null hypotheses. However, the testing of a null hypothesis does not take place within a vacuum, and other factors must be taken into account. These other factors include 1) how many questions are being asked in a given research project, 2) how many other similar projects may be taking place elsewhere by other researchers, and most importantly, 3) what is the ratio of null relationships to actual relationships existing in a given area of inquiry.
To help illuminate the argument, Ioannidis (Wilson, 2016) asks readers to suppose there are 101 stones in a given field. Only one of them, however, contains a diamond (i.e., a true finding). Gratefully, we have at our disposal a diamond-detecting machine that advertises a 99% accuracy of detection (i.e., hypothesis testing using inferential statistics). That is, when the machine is placed over top a stone without a diamond in it, 99% of the time it will not light up. Only 1% of the time will it give us a false positive (or, Type I error). Further, imagine that after checking several stones and getting no reaction, the machine finally starts to flash with activity. What is the probability that this stone, if cracked open, will contain a diamond? We might initially suggest that there is a 99% chance. However, recall that there are 100 dud stones in this field. The machine, if functioning at a 1% false positive rate, will register, on average, 1 false positive if all stones are checked. This means, of the 101 stones in the field, two are likely to register as positive for the diamond (one false positive and one real positive). Therefore, there is only a 50% chance of finding a diamond when this particular stone is cracked open. This is a little disappointing.
Now, imagine a field that has several thousand stones in it; still only one of them containing a diamond. Do we see how in this situation even a false positive rate of 1% may lead persistent researchers to draw faulty conclusions far too frequently? One key factor here, which is impossible to answer, is the ratio of stones containing diamonds. As this ratio increases, the ratio of true positives to false positives will improve. However, how do researchers know ahead of time in what sort of “field” they are working? Herein lies a big problem, the unknown ratio of real to null findings in a given area of investigation. Knowing the detection equipment has a 1% false positive rate does not solve this problem.
Further, do we see how the repeated testing of several stones changes the meaning of the 1% false positive rate? If we were to walk up to a field of stones and just test one, then the false positive rate of 1% makes sense. However, as we test stone after stone, the probability that at least one of the dud stones will register as significant grows as we work our way across the field. Herein lies a second problem, the additive nature of the Type I error rate.
One way to combat these problems, in addition to valuing replication (see Box 7.2), is to publicly report non-significant findings. Only once researchers get a sense of how few “diamonds” there are in a field of inquiry can they begin to process what a supposed finding might mean. If the field of inquiry seems to be chock-full of effects and relationships, then a significant claim seems more likely to be an actual finding; but if the field has repeatedly been shown to be lacking meaningful findings, then a claim of significance should be interpreted with a great deal of suspicion. Unfortunately, despite the current reproducibility crisis, there seems to be little interest in creating publication opportunities for null findings. Until this happens, the Type I error problem is going to continue to bring a cloud of suspicion around claims of findings, especially those coming from new, previously unexplored, fields of inquiry.
Find this and other essays regarding “Is the Scientific Method Broken?” in the Nesselroade & Grimm textbook.
Freedman, D. H. (2010, November). Lies, damned lies, and medical science. The Atlantic, 306(11). Retrieved from https://www.theatlantic.com/magazine/archive/2010/11/lies-damned-lies-and-medical-science/308269/?single_page=true
Ioannidis, J. P. A. (August 1, 2005). Why most published research findings are false. PLoS Medicine, 2(8), e124. https://doi.org/10.1371/journal.pmed.0020124
Leek, J. T. & Jager, L. R. (2017). Is most published research really false? Annual Review of Statistics and its Applications, 4, 109-122.
Wilson, W. A. (2016, May). Scientific regress. First Things. Retrieved from https://www.firstthings.com/ article/2016/05/scientific-regress