(Essay found in Nesselroade & Grimm, 2019; pgs. 209- 210)
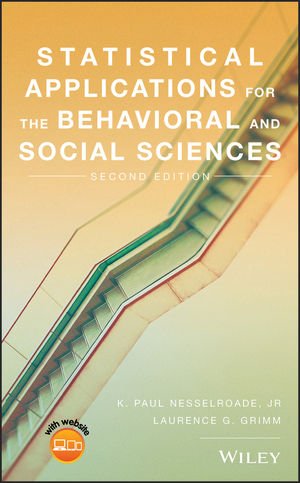
Programs found on the internet allow us actually to see how changing the sample size, mean, and the standard deviation of the population of raw scores change the resulting sampling distribution. Some of the ones recently found online include the StatKey Sampling Distribution for a Proportion program (www.lock5stat.com/StatKey/sampling_1_cat/sampling_1_cat.html), the Rice Virtual Lab in Statistics (onlinestatbook.com/stat_sim/sampling_dist/) and the Rossman/Chance Applet Collection (www.rossmanchance.com/applets/OneSample.html). There are others. A program that is quite flexible, however, is one created by Dr. Patrick Wessa (www.wessa.net/rwasp_samplingdistributionmean.wasp). In this program, we can input the number of replications we want to make (theoretically, sampling distributions involve the number of replications that equals the total number of samples of size n that are possible; often in the billions or more, so choose a number in the hundreds at least), the sample size (n), the mean of the population of raw scores, and the standard deviation of the population of raw scores. (It is recommended to leave the width and height of the chart as the given default values.) After imputing some values, click the “compute” button, wait for the program to run, and then scroll down to the second chart. Here we will see a graph reflecting the various sample means given our selected sample size, mean, and standard deviation.
By changing the values of the imputed numbers, we should be able to see the general principles of the sampling distribution concept as well as the claims of the Central Limit Theorem on display. For instance, if we increase the sample size, we should see the standard deviation of the sampling distribution (or, the standard error) tighten (and vice versa if we decrease the sample size). (If it does not look like it has tightened, check the values on the X axis to see if they were adjusted to better present the data.) If we change the value of the mean, the entire distribution should shift to be centered on the new mean. If we increase the standard deviation, the resulting sampling distribution should widen (and vice versa if we decrease the standard deviation). By exploring this program (or the others previously mentioned) we should be able to see that the mean of the means (µM) = the mean of the raw scores (µ), the standard error of the mean (σM) = the standard deviation of the raw scores divided by the square root of the sample size (σ/√n), and all sampling distributions approximate a normal distribution.
Find this and other similar side-bar discussions in the Nesselroade & Grimm textbook.