(Essay found in Nesselroade & Grimm, 2019; pgs. 79 – 80)
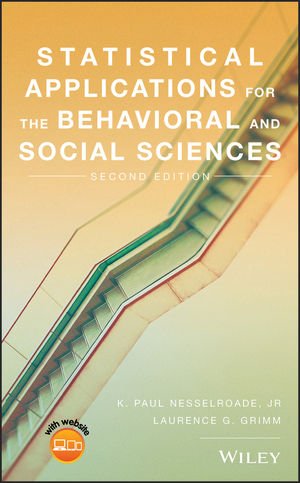
In Chapter 2 readers were introduced to a critical difference between ordinal scales and interval or ratio scales; the nature of the relationship between numerical values. Ordinal scales are a quantitatively organized series of categories, and as such, make no assumptions about the quantitative distance between these categories. Interval and ratio scales hold the intervals constant throughout the measure. In this chapter we learned that the concept of a deviation score is necessary to find a mean. A mean is defined as the point where the deviation scores sum to zero. Deviation scores, however, cannot be found for numbers on an ordinal scale; the intervals are not held constant. (It would be like suggesting first and third place finishers in a pie bake-off are equidistant from second. We have no reason to presume that.) Readers were also made aware of the ambiguity that surrounds how to interpret numbers generated by a Likert scale; scales which typically offer 5 to 11 options ranging from strongly disagree to strongly agree (usually with a neutral point in the middle) which are used to measure the amount of agreement people have with a given statement. The great debate is this: is it appropriate to generate means for Likert scale data?
It is very important because if we decide the answer is “no,” then we eliminate all statistical tests that make use of the concept of the mean. Much of the content in statistics textbooks is not applicable to data measured on an ordinal scale. An answer of “no” resigns us to use what are called nonparametric tests. (Chapters 17 and 18 in this textbook are devoted to nonparametric tests.) These tests are less powerful (a concept we will explore in Chapter 11), and therefore, less likely to help us find meaningful differences between groups.
The conservative approach is to argue that Likert scales have no way to determine constancy between values and should therefore be considered ordinal. As a result, data gathered using Likert scales must be analyzed nonparametrically; end of discussion. Others argue that the line between ordinal and interval is rather vague, some even calling it ‘fuzzy’ (e.g., Abelson, 1995). If, they argue, the data from a Likert scale takes-on the shape of a normal distribution, and if there are a good number of options for the respondent to choose from, then the data can be considered ‘normal’ or ‘sufficiently close’ to normal and analyzed with more standard statistical techniques.
We do not aim to settle the debate here, but merely raise it as an important issue. Perhaps it will be a good class discussion topic. As we think about this issue, keep in mind some recommendations made by Karen Grace-Martin, a specialist in data analysis. They are paraphrased below (Grace-Martin, 2008):
- Realize the difference between a Likert-type item and a Likert Scale. A Likert Scale is actually made up of many items. Collectively, they attempt to provide a measure of the attitude in question. Many people, however, use the term Likert Scale to refer to a single item.
- Proceed with caution. Look at the particulars of our Likert scale data. Would treating it as interval data influence our conclusions? The fact that everyone else is treating it as interval data is not sufficient justification in-and-of itself.
- At the very least, insist a) that the item have at least 9 points, b) that the underlying concept be continuous, and c) that there be some indication that the intervals between the values are approximately equal. Make sure the other statistical assumptions for the test are met.
- When we can, run the nonparametric equivalent to our test. If we get the same results, we can be confident about our conclusions.
- If we do choose to use Likert data in a parametric procedure, make sure we have particularly strong results before making a claim.
- Consider the consequences of reporting inaccurate results. Is the analysis going to be published? Will it be used by others to make decisions?
The hope here is less about bringing readers to some desired position and more about helping students develop an appreciation for some of the more subtle and yet important debatable issues related to data analysis. How we understand what the value of our scores mean is critical, requiring us to first figure out the type of scale being used. And if we find that we are using Likert data; well then, welcome to the great debate!
Find this and other similar side-bar discussions in the Nesselroade & Grimm textbook.
Abelson, R.P. (1995). Statistics as Principled Argument. Hillsdale, NJ: Erlbaum.
Grace-Martin, K. (2008). Can Likert Scale data ever be continuous? [The Analysis Factor]. Retrieved from http://www.theanalysisfactor.com/can-likert-scale-data-ever-be-continuous/